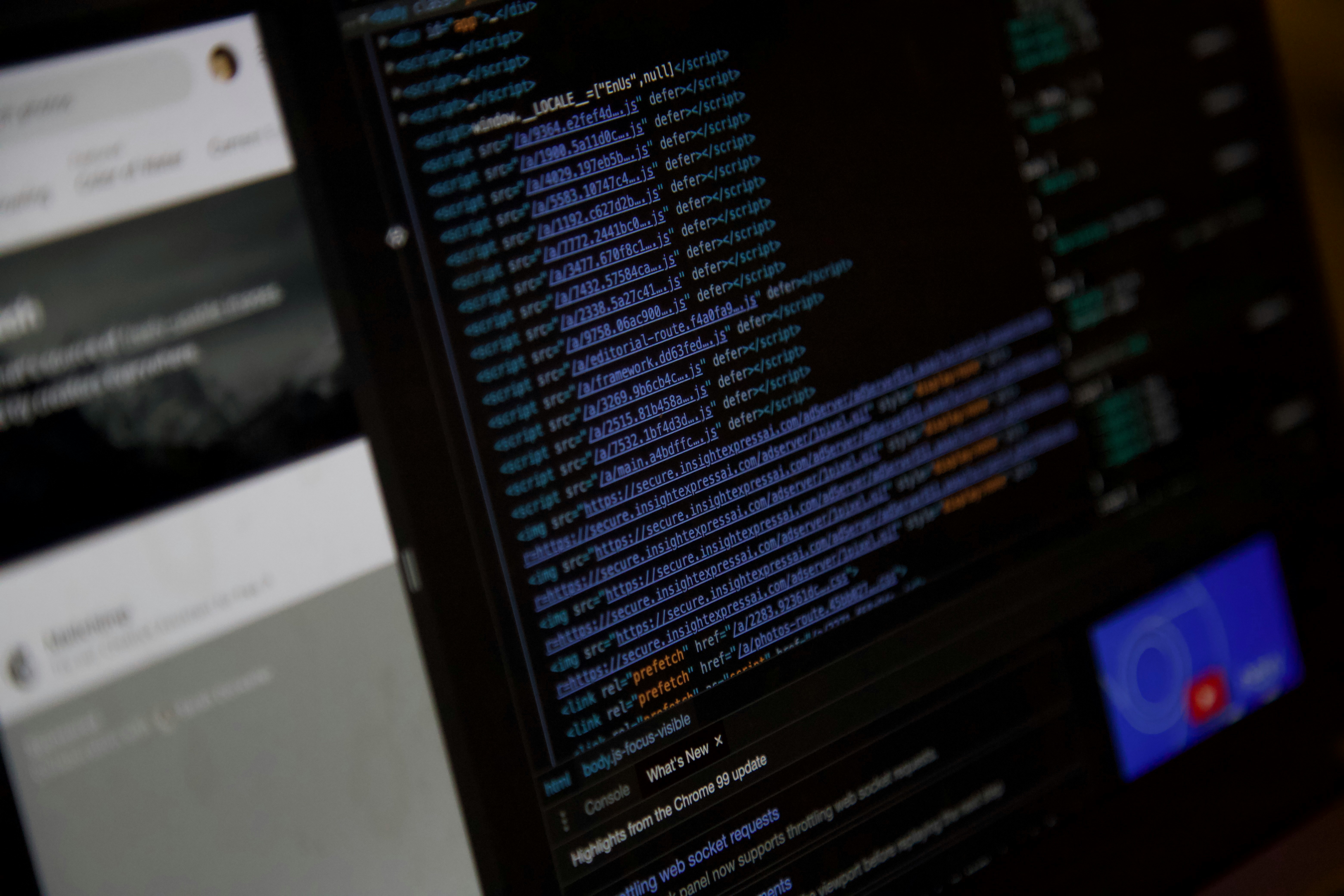
Robotics Programming Languages for Job Seekers: Which Should You Learn First to Launch Your Robotics Career?
From autonomous vehicles and warehouse automation to surgical robots and companion drones, the field of robotics is advancing at an unprecedented pace. This rapid innovation has created a surging demand for talented robotics engineers, software developers, and researchers capable of writing robust, efficient code to power these systems. If you’re exploring opportunities on www.roboticsjobs.co.uk, one question stands out: Which programming language should you learn first for a successful robotics career?
The answer isn’t one-size-fits-all. Robotics encompasses a broad range of tasks—real-time embedded development, high-level AI, motion planning, perception—and each domain has its own language preferences and ecosystem. Whether you’re interested in designing hardware-level control loops, building autonomous navigation software, or developing vision-based AI, selecting the right language (or combination of languages) is key to your success. In this article, we’ll:
Highlight the top programming languages used in modern robotics.
Break down pros, cons, and industry applications for each language.
Offer a hands-on beginner’s project to cement your learning.
Provide critical resources and tips to help you stand out on www.roboticsjobs.co.uk and beyond.
The Robotics Programming Landscape
Robotics integrates software, hardware, mechanical systems, and electronics into complex machines that sense, think, and act. As a result, robotics software must address:
Real-Time Control: Precisely coordinating motors, sensors, and feedback loops.
Reliability: Minor errors can cause physical damage or endanger safety.
Performance: Some tasks, like advanced path planning or 3D vision, demand efficient and optimised code.
Interoperability: Robotics systems often incorporate multiple libraries and frameworks, from low-level firmware to high-level AI modules.
Each programming language has its place in this diverse ecosystem, so let’s explore the major players.
1. C and C++
Overview
C and C++ are arguably the bedrock of robotics. They’re widely used for low-level control, real-time operations, and performance-critical applications. The Robot Operating System (ROS), one of the most popular robotics middleware platforms, is largely built in C++, with C++-based APIs to manage nodes, messaging, and other essential functionalities.
Key Features
Memory Control: Direct pointers and manual allocation let you fine-tune real-time constraints.
Performance: Compiled code runs quickly, vital for tasks like motion planning and embedded microcontroller code.
Community Support: Decades of usage in embedded systems, advanced robotics libraries (e.g., OpenCV for vision).
Pros
Determinism: Ideal for safety-critical or real-time loops.
Widely Supported: Many microcontrollers, sensors, and robotics frameworks provide C/C++ APIs.
Scalability: Use C++ for advanced path planning, perception, or integration with robotics frameworks like ROS or OROCOS.
Cons
Complexity: Debugging pointer arithmetic, memory leaks, or concurrency bugs can be challenging.
Steep Learning Curve: Beginners might find C++ syntax overwhelming, especially modern features (templates, lambdas).
Longer Development Times: More boilerplate code than higher-level languages like Python.
Who Should Learn C/C++ First?
Embedded Robotics Engineers focusing on real-time microcontrollers (Arduino, STM32, etc.).
Developers Working with ROS for advanced robotics solutions or custom hardware integrations.
Performance-Critical Applications (drone flight control, manipulator motion planning, SLAM algorithms).
2. Python
Overview
Python has soared in popularity across many fields, including robotics. While C/C++ handle low-level tasks, Python excels at rapid prototyping, scripting, and high-level behaviour. Python also integrates seamlessly with machine learning frameworks, making it crucial for AI-driven robotics (computer vision, deep learning–based planning).
Key Features
High-Level API: Easy for scripting complex robot behaviour, orchestrating tasks, or prototyping algorithms.
Bindings to Lower-Level Libs: Many robotics libraries written in C/C++ provide Python wrappers for convenience.
Machine Learning Synergy: Libraries like TensorFlow, PyTorch, and OpenCV (Python bindings) streamline AI in robotics.
Pros
Simplicity: Intuitive syntax, quick development cycles—especially useful for educational or research prototypes.
ROS Support: ROS includes a robust Python interface (rospy).
Huge Ecosystem: Tools for data analysis, image processing, neural networks, or IoT integration.
Cons
Performance: Interpreted code is slower than compiled equivalents; advanced real-time tasks may require custom C/C++ modules.
Dependency Management: Virtual environments, version conflicts, or packaging can be tricky in robotics deployments.
Not Ideal for Hard Real-Time: Python’s garbage collection and overhead hamper deterministic timing.
Who Should Learn Python First?
Robotics Students and Researchers building rapid prototypes, educational robots, or AI-driven modules.
Software Engineers focusing on high-level logic, sensor fusion, or simulation in frameworks like ROS.
Teams Integrating AI/ML for perception, planning, or predictive maintenance in robotics.
3. ROS (Robot Operating System)
Overview
Strictly speaking, ROS isn’t a programming language; it’s an open-source middleware providing essential tools, libraries, and conventions for building complex robot software. However, ROS is so central to modern robotics that familiarity with its structure and APIs is almost a requirement for many robotics positions.
Key Features
Node-Based Architecture: Define separate modules (nodes) for sensor reading, motion planning, and actuation, each communicating via topics and services.
Language Agnostic: Official support for C++ (roscpp) and Python (rospy), with experimental support for other languages.
Rich Ecosystem: Thousands of packages for localisation (SLAM), path planning, manipulation, vision, and more.
Pros
Modularity: Encourages clean separation of concerns across multiple robot subsystems.
Community: Large user base, widely used in research labs and industrial contexts alike.
Simulation Tools: Integration with Gazebo, rviz, and other simulation/visualisation environments for rapid testing.
Cons
Learning Curve: Understanding nodes, topics, services, tf transformations, and build systems (catkin, colcon) can be daunting.
Version Fracture: ROS1 vs. ROS2—some libraries or drivers may not be fully migrated.
Not for Bare-Metal: Typically runs on a Linux OS with moderate computing power, so microcontroller-level robotics might not rely on ROS.
Who Should Learn ROS First?
Anyone Aiming for Modern Robotics Roles in research labs, start-ups, or large robotics companies.
Developers Building Complex Systems with multiple sensors, actuators, and algorithms needing modularity.
Teams Seeking Rapid Development with pre-built packages for SLAM, navigation, manipulation, etc.
4. Rust
Overview
Rust is a systems-level language emphasising memory safety and performance—two critical aspects of robotics software. While not as entrenched in robotics as C/C++ or Python, Rust is gaining traction for embedded and real-time applications because it eliminates entire classes of memory-related bugs and can deliver near-C++ performance.
Key Features
Ownership and Borrowing: Prevents null pointer dereferences, data races, or use-after-free errors at compile time.
Concurrency: Built-in abstractions (threads, channels, async) for parallel or real-time tasks in robotics.
Growing Ecosystem: Gradual adoption in robotics circles (e.g., the
rclrs
client library for ROS2 in Rust).
Pros
Memory Safety: Helps avoid catastrophic crashes or vulnerabilities in safety-critical systems.
Performance: Comparable to C++ for CPU-intensive tasks like path planning, sensor fusion.
Modern Features: Pattern matching, generics, macros, plus an integrated package manager (Cargo) for simpler builds.
Cons
Steep Learning Curve: Borrow checker and ownership rules can be tricky, especially for novices.
Less Established in Robotics: Fewer libraries and pre-built solutions than in C++ or Python, though this is changing.
Longer Compile Times: More advanced features and compile-time checks often slow down build iteration.
Who Should Learn Rust First?
System-Level Roboticists seeking memory safety for real-time or embedded code.
Engineers Tired of debugging pointer issues or concurrency bugs in C/C++.
Early Adopters comfortable experimenting with emerging ecosystems like ROS2 Rust.
5. Java
Overview
While not as popular in robotics as C++ or Python, Java finds use in certain industrial or enterprise robotic applications—particularly where JVM-based architectures or large-scale software engineering approaches are standard. Libraries like ArduPilot or certain Android-based robotics platforms also leverage Java.
Key Features
Platform Independence: JVM can run on diverse hardware, from full Linux boxes to embedded boards like the Raspberry Pi.
Extensive Library Ecosystem: Tools for networking, concurrency, and UI.
Enterprise Integration: Some industrial robots or warehouse automation systems might rely on Java for data ingestion, monitoring, or analytics.
Pros
Mature Tooling: IDEs (IntelliJ, Eclipse) help manage large codebases.
Stability: Java’s garbage collection and memory management are well-tested (though not real-time).
Cross-Platform: Write once, run anywhere, beneficial for distributed robotic systems.
Cons
Less Real-Time Friendly: Garbage collection can cause unpredictable pauses, which can be problematic for time-critical loops.
Verbosity: More boilerplate code than Python, Rust, or modern C++.
Smaller Robotics Community: Fewer open-source projects for advanced robotics tasks, though some do exist (e.g., Pi4J for Raspberry Pi).
Who Should Learn Java First?
Engineers in Enterprise Environments bridging robotics solutions with large-scale data or infrastructure.
Developers Creating Android-based robotics or companion apps for robot interfaces.
Those Familiar with Java from other domains wanting to extend existing solutions to robotics.
6. MATLAB
Overview
MATLAB is a staple in robotics research, simulation, and algorithm development—especially for control systems, signal processing, and robot kinematics. Although less common in final production code, MATLAB’s robust toolboxes (e.g., Robotics System Toolbox, Simulink) are widely used in academia and advanced R&D labs for quick prototypes or advanced modelling.
Key Features
High-Level Scripting + Toolboxes: Specialised libraries for robotics, control engineering, and sensor fusion.
Simulink Integration: Visual block diagrams for real-time control system design—common in autopilot or manipulator control.
Extensive Documentation: Clear, well-structured references for algorithms from kinematics to advanced state estimation.
Pros
Ideal for Rapid Prototyping: Quickly implement, test, and tune complex control algorithms.
Visual Tools: Simulink’s block diagrams are intuitive for mechanical or electrical engineers.
Rich Ecosystem: Toolboxes for everything from path planning to 3D visualisation in robotics.
Cons
Licence Costs: MATLAB is proprietary, which can be expensive for start-ups or small labs.
Limited Production Use: Many teams rewrite MATLAB prototypes in C++/Python for deployment.
Learning Curve: The environment differs from standard text-based development, requiring time to master.
Who Should Learn MATLAB First?
Academic Researchers focusing on algorithm development, simulation-based approach, or advanced controls.
Control Systems Engineers designing servo loops, motor control, or multi-DOF kinematic solutions.
Labs or Companies that can afford licensing and rely on MATLAB for rapid iteration.
Other Notable Mentions
Lua: Sometimes used for scripting in robotics frameworks or small embedded systems.
JavaScript/TypeScript: For web dashboards or controlling robots via browser-based interfaces.
Go: Gaining traction in microservices for cloud robotics, though less established in real-time or embedded contexts.
Choosing the Right Language for Your Robotics Career
When scanning roles on www.roboticsjobs.co.uk, note the job requirements: some might emphasise ROS and C++ for industrial manipulator arms, others might need Python for AI-based perception, while certain start-ups could champion Rust for next-generation embedded solutions. Consider:
Focus Area
Low-Level Embedded: C/C++ or Rust if you want modern memory safety.
High-Level AI and Prototyping: Python is king, with possible MATLAB in academic contexts.
Middleware & Architecture: ROS knowledge is practically essential for complex robot software.
Enterprise/Android: Some roles rely on Java or .NET-based solutions.
Performance vs. Prototyping
Real-time or hardware-bound tasks typically require C++ or Rust.
Quick experimentation often suits Python or MATLAB.
ROS can be used with either C++ or Python, depending on performance needs.
Existing Skill Set
If you have a background in embedded systems, picking up C/C++ is natural.
If you’re from a machine learning or data science environment, Python will be comfortable.
Keen on advanced concurrency or modern systems? Explore Rust.
Industry Trends
ROS2 is adopting more real-time features and is increasingly popular.
Rust is slowly gaining acceptance for safety-critical robotics.
Simulation Tools (Gazebo, Webots, Isaac Sim) are mostly C++ or Python-based.
Many robotics engineers ultimately become polyglots, using C++ for real-time modules, Python for AI and scripting, and ROS to glue it all together.
A Simple Beginner Project: ROS-Based Turtle Simulation (Python)
To practise fundamental robotics concepts, try this small ROS project—using Python (rospy) to control the turtlesim package. Turtlesim is a built-in simulator in ROS that displays a turtle on screen, which you can move with velocity commands.
Steps
Set Up ROS
Install ROS (e.g., ROS Noetic on Ubuntu 20.04).
sudo apt-get install ros-noetic-ros-tutorials ros-noetic-turtlesim
Source your ROS setup script:
source /opt/ros/noetic/setup.bash
.
Launch Turtlesim
bash
CopyEdit
rosrun turtlesim turtlesim_node
Create a Python Script
python
CopyEdit
# turtle_controller.py import rospy from geometry_msgs.msg import Twist def move_turtle(): rospy.init_node('turtle_controller', anonymous=True) pub = rospy.Publisher('/turtle1/cmd_vel', Twist, queue_size=10) rate = rospy.Rate(1) # 1 Hz twist = Twist() twist.linear.x = 2.0 # Move forward twist.angular.z = 1.0 # Slightly turn while not rospy.is_shutdown(): pub.publish(twist) rate.sleep() if __name__ == '__main__': try: move_turtle() except rospy.ROSInterruptException: pass
Run Your Script
In a new terminal (with ROS sourced):
bash
CopyEdit
rosrun YOUR_PACKAGE_NAME turtle_controller.py
The turtle in the simulator should move forward while slowly turning in circles.
Extend the Project
Subscribe to /turtle1/pose to get the turtle’s position, implementing a loop that stops the turtle after a certain distance.
Add keyboard input or a simple algorithm to move the turtle in shapes.
Explore other ROS topics or services (like /spawn, /kill) to spawn multiple turtles and control them.
This exercise demonstrates how ROS nodes communicate via topics, letting you control a robot (or a simulated turtle) with velocity commands. The C++ equivalent code is similar, except you’d compile it with catkin or colcon. This small introduction paves the way for more sophisticated robots in simulation or real hardware.
Essential Tools, Ecosystem, and Career Resources
Whether you choose C++, Python, Rust, or another language, your robotics journey will involve these common tools and frameworks:
Hardware
Microcontrollers (Arduino, STM32) or Single-Board Computers (Raspberry Pi, Jetson) for embedded robotics.
Sensors: LiDAR, depth cameras, IMUs, contact sensors.
Actuators: Servo motors, stepper motors, linear actuators.
Simulation & Visualisation
Gazebo: 3D multi-robot simulator integrated with ROS.
RViz: Visualises sensor data, robot state, and environment in ROS.
Webots, Ignition: Alternative robotics simulators.
Control & Planning
MoveIt!: ROS-based motion planning for manipulators and arms.
Navigation Stack: SLAM, path planning, and obstacle avoidance in ROS.
Autoware: Autonomous driving stack (for vehicles and mobile robots).
DevOps & Deployment
Version Control: Git, GitHub, GitLab for collaborative robotics software.
Continuous Integration: Tools like Jenkins or GitHub Actions for automated builds (especially crucial for large codebases).
Docker: Containerise and share robotics environments for consistent development setups.
Communities & Conferences
www.roboticsjobs.co.uk: Specialised job board for robotics roles in the UK.
ROS Discourse / ROS Answers: Q&A forums for ROS-related topics.
Conferences: ICRA, IROS, ROSCon, smaller local meetups for networking.
Conclusion
The robotics domain is broad, multidisciplinary, and continually evolving—making it an exciting and rewarding career path for engineers who love hands-on innovation. C/C++ remain essential for embedded control and real-time performance, Python excels at AI and high-level scripting, while ROS ties it all together as the de facto robotics middleware. For those seeking cutting-edge concurrency or memory safety, Rust is a promising newcomer. Meanwhile, MATLAB supports advanced algorithm prototyping, and Java retains a foothold in enterprise-oriented robotic systems.
Rather than limiting yourself to a single language, many successful roboticists learn a combination: using C++ (or Rust) for time-critical loops, Python for sensor fusion or AI modules, and ROS as the unifying framework. Regardless of your path, you’ll benefit from an understanding of hardware basics, control theory, and software engineering best practices. By choosing the right programming language based on your goals and following up with hands-on projects, you’ll make yourself a top candidate for roles at www.roboticsjobs.co.uk and shape the future of robotics—be it in healthcare, logistics, manufacturing, or beyond.